Synthetic Genetic Arrays – Personal Robotics for Omics with High-Density Yeast Arrays
Charles Boone¹, Amy Tong¹, Pawel Kusan², Harry Singer³, and Carl Singer³
¹Boone Lab, University of Toronto, Toronto, Canada M56 1L6. ²Engineering Services Inc., Toronto, Canada, M5R 2J7. ³Singer Instrument Co. Ltd., Roadwater, Somerset, TA23 ORE, UK.
Summary
Synthetic Genetic Array (SGA) analysis involves the manipulation of high-density yeast arrays to identify synthetic genetic interactions. In a typical SGA screen, a query strain is systematically crossed to an ordered array of deletion mutants, such that the meiotic progeny harbouring both gene mutations can be assayed for growth defects. Identifying these interactions allows for the construction of large genetic networks, which reveal functional dependency and pathway redundancy in yeast.
Principles of SGA
SGA offers an approach for the systematic construction of double gene deletion mutants and enables global analysis of genetic interactions [1,2]. Below are the steps involved in a SGA screen [3] :
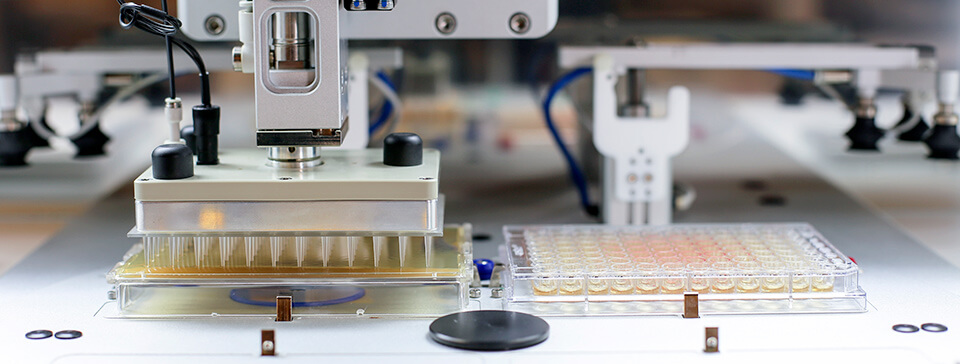
The ROTOR+ is the fastest and most powerful colony manipulation robot in the world. It is essential for any large-scale SGA studies.
- A MATα strain with a strain background of MFA1pr-HIS3, can1, and lyp1 is used to construct a query deletion mutant linked to a nourseothricin (clonNAT)-resistance marker, natMX.
- The query mutant is then crossed to an ordered array of MATa mutants, each carrying a gene deletion linked to the geneticin (G418)-resistance marker, kanMX (Figure 1A).
- The resulting heterozygous diploids are selected on a medium that contains clonNAT and G418 (Figure 1B).
- Then diploids are transferred to a medium that contains reduced levels of carbon and nitrogen to induce the sporulation of haploid meiotic progeny (Figure 1C).
- Spores are then transferred to medium that lacks histidine and contains canavanine and thialysine to select for MATa meiotic progeny (Figure 1D).
- MATa meiotic progeny containing both gene deletions is selected on a medium that lacks histidine and contains canavanine, thialysine, clonNAT, and G418. Double mutants that grow on such a medium are then scored for growth fitness (Figure 1E).
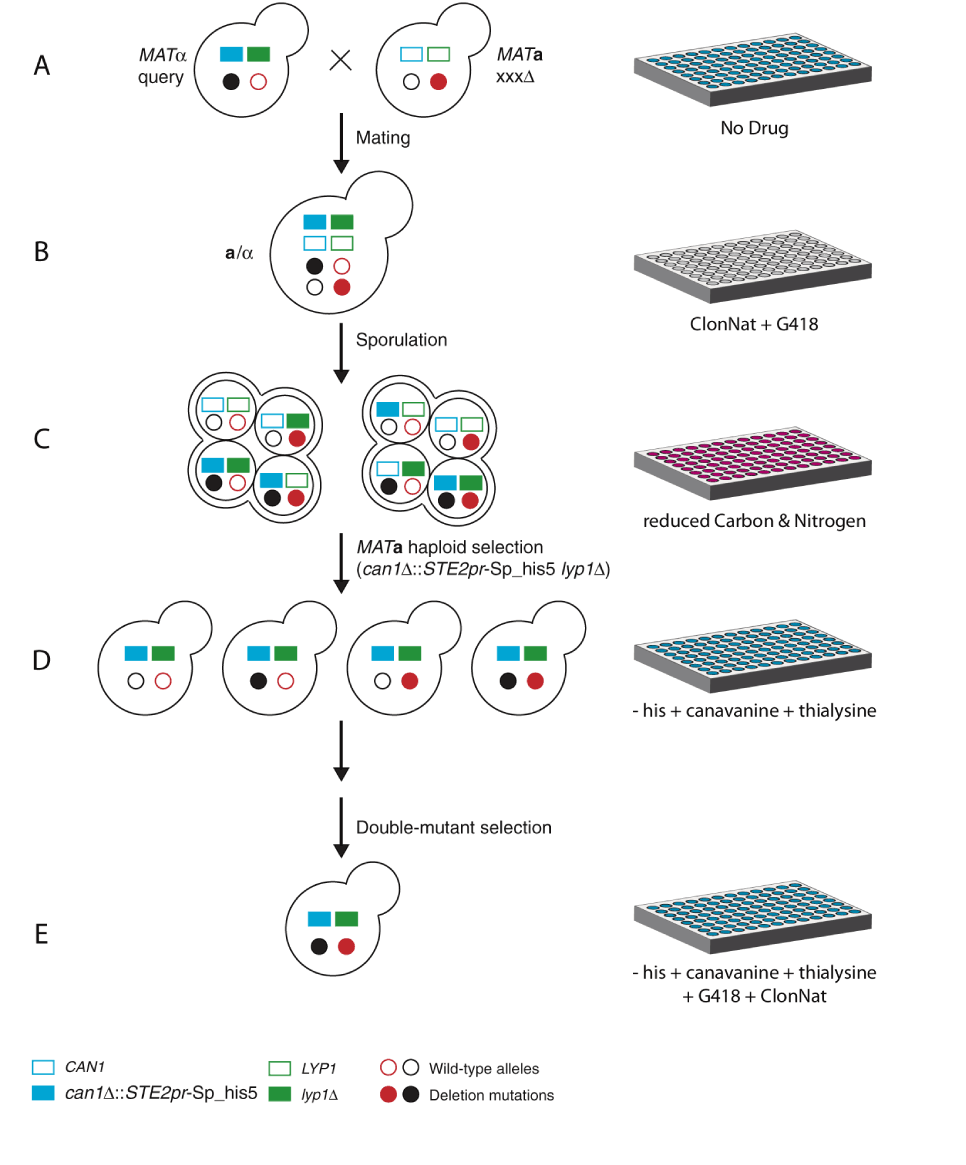
Figure 1: Principles of SGA. See text for details. (Adapted from Costanzo and Boone, 2009)
The theory behind genetic interactions
If two genes are functionally unrelated, the double mutant containing these two gene mutations would exhibit a phenotype that is approximately the product of the phenotypes of the individual mutants.
However, when mutations in two gene products produce a phenotype that deviates from each gene’s individual effect, these two genes exhibit a genetic interaction. There are two general categories of genetic interactions: aggravating interactions and alleviating interactions (Figure 2A).
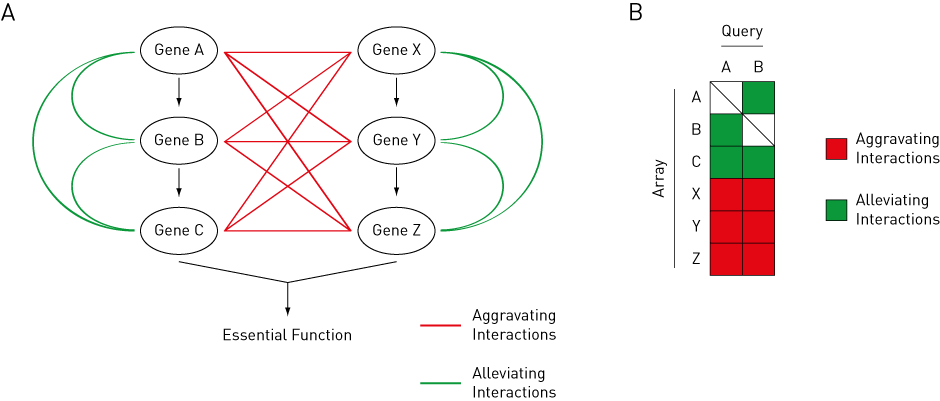
Figure 2: Theory behind genetic interactions. Aggravating interactions are shown in red, while alleviating interactions are shown in green. (A) Pathway ABC and XYZ both contribute to an essential function in a cell. Gene A shows aggravating interactions with Gene X, Y, and Z, but shows alleviating interactions with Gene B and C. (B) Gene A and B show similar genetic interaction profiles to each other.
An aggravating (or negative) interaction occurs when a double mutant exhibits a phenotype that is more severe than expected from the phenotypes of the individual mutants. This type of interaction indicates that the gene products function in redundant parallel pathways, and highlights the robustness of the molecular network in tolerating genetic variations [4].
An alleviating (or positive) interaction occurs when a double mutant exhibits a phenotype that is less severe than expected from the phenotypes of individual mutants. This type of interaction indicates that the gene products operate in concert or in series within the same pathway [4].
Genes that function in the same pathway tend to have similar genetic interaction profiles. Therefore, identifying genetic interactions can reveal functional relationships between genes and biological pathways [4] (Figure 2B).
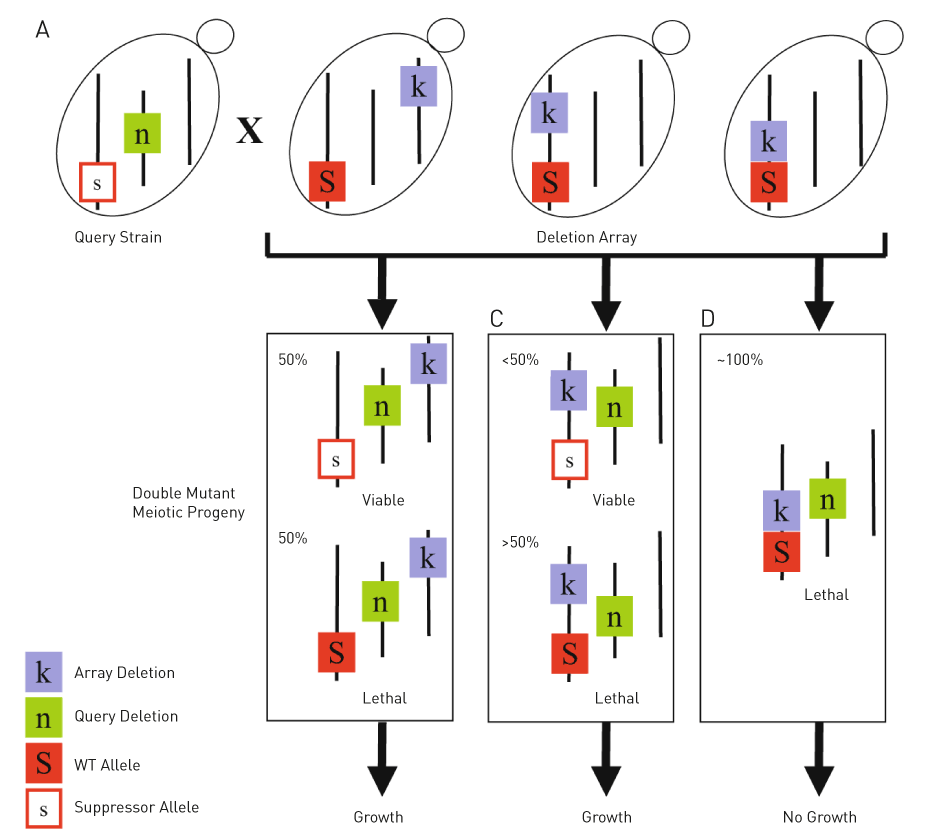
Figure 3: Mapping of genetic suppressors using SGAM. (A) In this example, the lethality associated with the query gene deletion (n, green box) is suppressed by a mutation of an unknown gene (s, open red box). Using SGA, this query strain is crossed to a genome-wide gene deletion array (k, purple box), and double mutant meiotic progenies are selected. (B) If the suppressor gene is located on a different chromosomal locus than the array deletion gene, the segregation of these two genes will occur independently of each other. Therefore, half of the double mutants will contain the suppressor and survive, while the other half will contain the wild-type gene (S, filled red box) and die. (C) However, if the suppressor gene is located on the same chromosome as the array deletion gene, these two genes are linked and the segregation of the array deletion gene will tend to follow the wild-type gene. Therefore, only a minority of double mutants will contain the suppressor and survive. (D) If the wild-type version of the suppressor and the array deletion gene are located next to each other, these two genes are tightly linked and almost always segregate with each other. Therefore, no double mutant will contain the suppressor gene. Consequently, no colony will survive. (Adapted from Costanzo and Boone, 2009)
Applying SGA to Functional Studies
SGA analysis enables the genome-wide screening of synthetic lethal or synthetic sick interactions, which are examples of aggravating interactions. Synthetic interactions reveal functional relationships between genes whose products buffer each other or impinge on a common essential pathway. Therefore, SGA analysis sheds light on the function of genes and permits the identification of novel components of specific pathways [1].
SGA analysis can be applied to the high-resolution mapping of genetic suppressors (Figure 3). A suppressor is a mutation occurring elsewhere in the genome that alleviates the growth defect of the query mutant. This application, termed SGAM (SGA mapping), is based on the principle that the frequency of meiotic recombination, which is the basis of creating double mutants in SGA, reduces as chromosomal locations of two genes get closer [5]. Therefore, a set of genes that is closely located, or “linked,” to the suppressor gene would appear synthetic lethal/sick with the query mutation. Identifying genetic suppressors is useful because alleviating interactions reveals genes whose products function in the same complex or pathway [6].
Suppressor Mapping
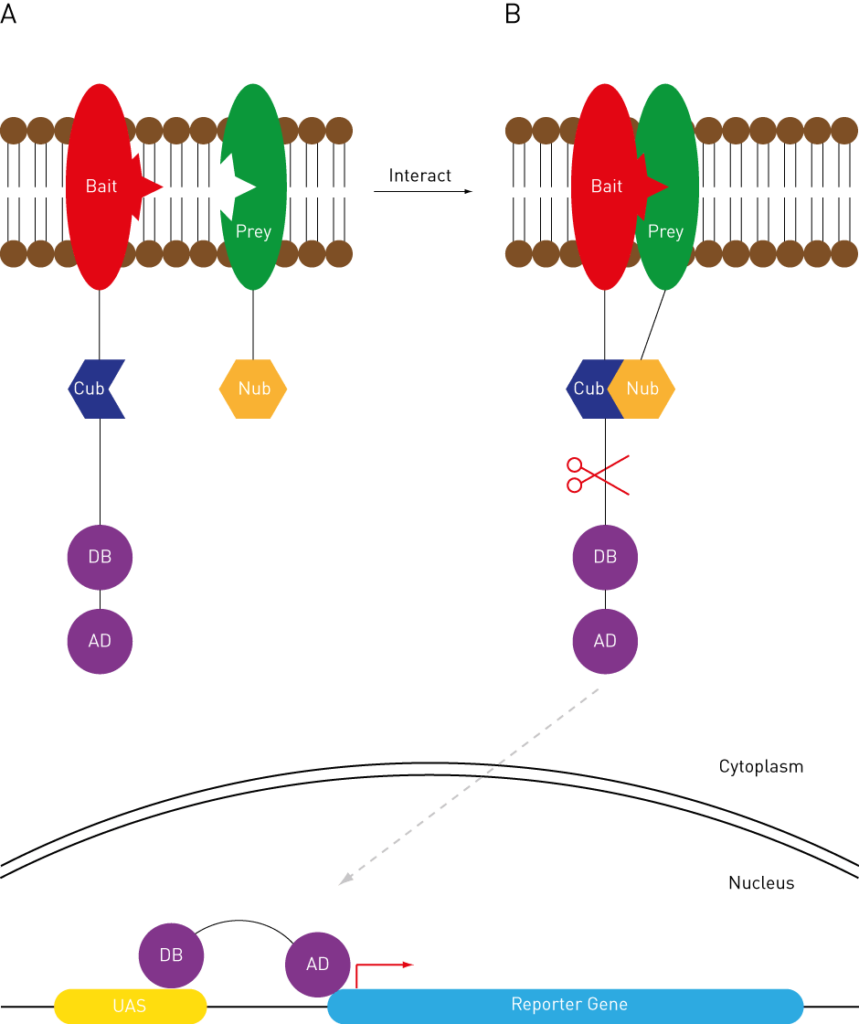
Library Collections
In addition to the deletion collections [7,8], there are many other genome-wide libraries available in the yeast community. A sample of the available libraries includes arrays of the Tet-promotor [9] and transposon [10] mutants, genes tagged with TAP (tandem affinity purification) [11] and GFP (green fluorescent protein) [12], and strains containing Y2H (yeast 2-hybrid) [13] and GST (glutathione S-transferase) [14] fusion plasmids.
This collection of library arrays extends the applications of the SGA methodology to other forms of interactions, including dosage lethality and higher-order genetic interactions [3], thereby allowing scientists to comprehensively decipher biological networks in yeast.
Working with Libraries
High-throughput SGA screening can be done quickly and easily using automated robotics, such as the Singer ROTOR+, which can rapidly pin high-density arrays of yeast as well as facilitate strain mating, sporulation, and selection. In addition, the ROTOR+ screening robot provides an automated platform to simultaneously produce multiple experimental replicates.
SGA Applications
Parkinson’s Disease Genes VPS35 and EIF4G1 Interact Genetically and Converge on α-Synuclein Abstract (Dhungel N, Eleuteri S, Li L-B, Kramer NJ, Chartron JW, Spencer B, Kosberg K, Fields JA, Stafa K, Adame A, Lashuel H, Frydman J, Shen K, Masliah E, Gitler AD)
Parkinson’s disease (PD) is a common neurodegenerative disorder. Functional interactions between some PD genes, like PINK1 and parkin, have been identified, but whether other ones interact remains elusive. Here we report an unexpected genetic interaction between two PD genes, VPS35and EIF4G1. We provide evidence that EIF4G1 upregulation causes defects associated with protein misfolding. The expression of a sortilin protein rescues these defects, downstream of VPS35, suggesting a potential role for sortilins in PD. We also show interactions between VPS35, EIF4G1, and α-synuclein, a protein with a key role in PD. We extend our findings from yeast to an animal model and show that these interactions are conserved in neurons and in transgenic mice. Our studies reveal unexpected genetic and functional interactions between two seemingly unrelated PD genes and functionally connect them to α-synuclein pathobiology in yeast, worms, and mouse. Finally, we provide a resource of candidate PDgenes for future interrogation.